Windows 11 Recall: The Privacy Feature That Sparked a Digital Firestorm
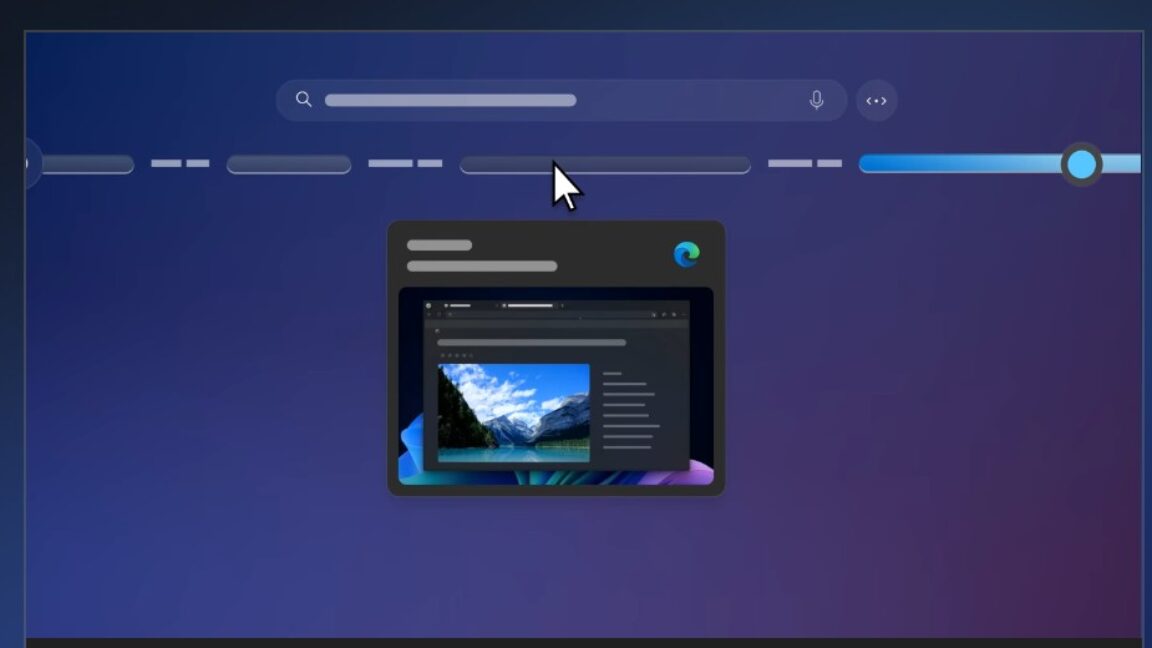
The shadows of a previous misstep continue to loom over the latest iteration of the AI data-scraping feature, serving as a stark reminder of the challenges tech companies face when introducing innovative technologies.
When the feature was first unveiled, it encountered significant turbulence, with critics and users alike raising serious concerns about its data collection methodology and potential privacy implications. Those initial stumbles have cast a long-lasting impression that the development team is now working diligently to overcome.
The new version represents a comprehensive effort to address the shortcomings of its predecessor. Engineers have meticulously redesigned the feature's architecture, implementing more robust privacy safeguards and transparent data collection protocols. Their goal is not just to improve functionality, but to rebuild user trust that was eroded during the original launch.
Despite these improvements, the feature still faces an uphill battle in public perception. The memory of its botched debut continues to influence how potential users and industry observers view its current capabilities. This underscores the critical importance of first impressions in the rapidly evolving landscape of artificial intelligence technologies.
As the team pushes forward, they remain committed to demonstrating that the feature has genuinely transformed, offering a more responsible and user-centric approach to data scraping.