Beyond Bigger: Why More AI Isn't Always Smarter, Reveals Meta's Top Scientist
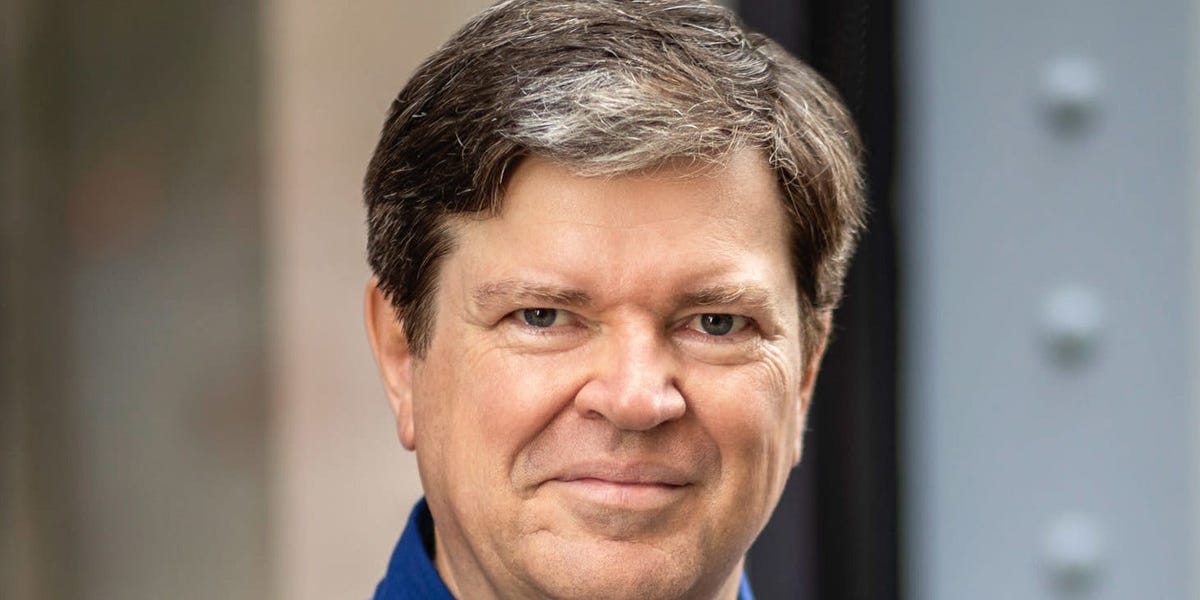
In a bold challenge to the current AI development paradigm, Yann LeCun, Meta's chief AI scientist, is pushing back against the prevailing notion that larger AI models are inherently superior. LeCun argues that the future of artificial intelligence lies not in simply scaling up model size, but in fundamentally reimagining how these systems are trained.
The renowned AI expert suggests that true intelligence requires a more nuanced approach—one that moves beyond the current trend of creating increasingly massive neural networks. Instead, LeCun advocates for smarter, more efficient training methodologies that can help AI systems develop more meaningful and adaptable capabilities.
By questioning the "bigger is better" mantra that has dominated recent AI research, LeCun is signaling a potential shift in how we think about artificial intelligence development. His perspective challenges tech giants and researchers to focus on quality and intelligent design rather than simply increasing computational complexity.
As the AI landscape continues to evolve, LeCun's insights offer a provocative roadmap for creating more sophisticated and genuinely intelligent artificial systems that can learn and adapt more effectively.