Behind the Hype: Google Gemini's Pokémon Breakthrough Falls Short of Expectations
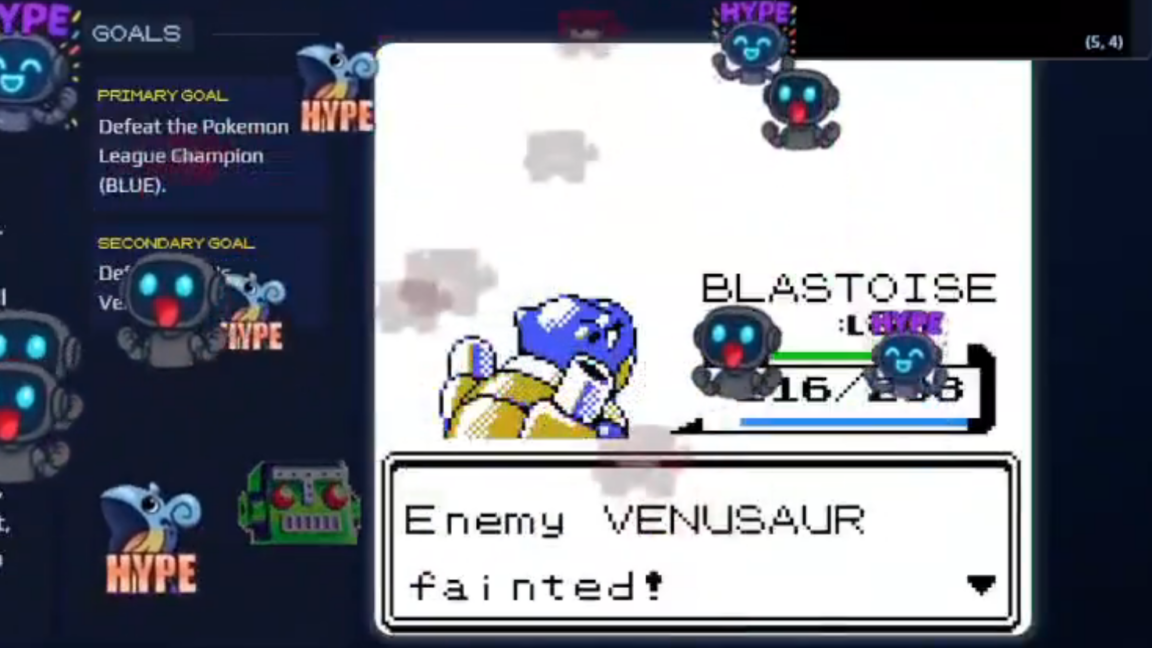
The breakthrough in the model's performance can be largely attributed to an innovative external "agent harness" that dramatically enhanced its capabilities. This sophisticated support mechanism played a crucial role in unlocking the model's potential, providing strategic guidance and computational assistance that elevated its overall effectiveness.
By leveraging this external agent framework, researchers were able to create a more dynamic and responsive system that could adapt and learn with unprecedented precision. The agent harness acted as a sophisticated scaffolding, enabling the model to process complex information more efficiently and generate more nuanced outputs.
The integration of this external support system represents a significant advancement in artificial intelligence, demonstrating how strategic external assistance can transform computational models from merely functional to truly exceptional. This approach highlights the potential of collaborative frameworks in pushing the boundaries of technological innovation.